CDS, Guidelines and Planning Overview
Issuer: openEHR Specification Program | |
---|---|
Release: PROC latest |
Status: TRIAL |
Revision: [latest_issue] |
Date: [latest_issue_date] |
Keywords: process, guidelines, GDL, planning, CDS, workflow, CPG |
© 2020 - 2022 The openEHR Foundation | |
---|---|
The openEHR Foundation is an independent, non-profit foundation, facilitating the sharing of health records by consumers and clinicians via open specifications, clinical models and open platform implementations. |
|
Licence |
|
Support |
Issues: Problem Reports |
Amendment Record
Issue | Details | Raiser, Implementer | Completed |
---|---|---|---|
PROC Release 1.6.0 |
|||
SPECPROC-45. Add Process component Overview document. |
T Beale |
||
GDL-oriented review. |
T Beale, |
30 Jan 2021 |
|
0.5.1 |
Improvements due to reviews. |
R Chen, |
15 Dec 2020 |
0.5.0 |
Initial writing. |
T Beale |
15 Dec 2020 |
Acknowledgements
1. Preface
1.1. Purpose
This document provides an overview of the openEHR PROC component specifications, including the openEHR Decision Logic Module (DLM) Language, openEHR Task Planning and Subject Proxy service.
The intended audience includes:
-
Standards bodies producing health informatics standards;
-
Academic groups using openEHR;
-
Solution vendors.
1.2. Related Documents
Prerequisite documents for reading this document include:
Related documents include:
1.3. Status
This document is in the TRIAL state. The development version of this document can be found at https://specifications.openehr.org/releases/PROC/latest/overview.html.
Known omissions or questions are indicated in the text with a 'to be determined' paragraph, as follows:
TBD: (example To Be Determined paragraph)
1.4. Feedback
Feedback may be provided on the process specifications forum.
Issues may be raised on the specifications Problem Report tracker.
To see changes made due to previously reported issues, see the PROC component Change Request tracker.
2. Introduction
2.1. openEHR Process and Planning Specifications
openEHR provides specifications for various models and languages to address the area of process and guideline-driven healthcare. These are as follows:
-
A plan definition formalism: the openEHR Task Planning specification (TP);
-
A guideline and rule language: the openEHR Decision Language specification (DL);
-
An expression language, which forms the basis for writing expressions and rules, defined by the openEHR Expression Language (EL);
-
A subject proxy service, which forms the bridge between Plans and Guidelines as consumers of subject data, and data sources: the openEHR Subject Proxy Service.
-
An underlying meta-model formalising information models, expression language and decision constructs, defined by the openEHR Basic Meta-Model;
Together these enable the authoring of care pathways, guidelines, executable care plans and in-application active forms. There is also an existing guideline language, Guideline Definition Language 2 (GDL2), which defines an earlier self-standing guideline format, on which some self-standing guidelines solutions are built. In some circumstances, such systems may be equivalently implemented in the future using TP/DL/EL instead of GDL2.
Many of the formal semantics of the DLM formalism comes from experience with existing GDL2 deployments.
2.2. Prior Art
The openEHR Task Planning specification is conceptually close to the YAWL language (Yet Another Workflow Language) (Hofstede, van der Aalst, Adams, & Russell, 2010), as well as to the OMG BPMN and CMMN standards, which support different flavours of workflow. Many ideas were adopted and/or adapted from YAWL, of which the only deficiencies are: being agnostic on rule language and data persistence (both of centra importance in healthcare IT), and no longer being actively maintained. The BPMN and CMMN standards have a weaker theoretical foundation, and were not originally conceived as being business analysis rather than executable formalisms; they also have only limited worker definition and allocation semantics, are weak on data persistence and have a rules expression formalism (DMN) that is relatively under-developed or tested in healthcare.
openEHR GDL2 and the more recent Decision Language may be compared to other languages developed in the health arena for expressing medical logic and rules, including HL7 Arden Syntax, Guideline Interchange Format (Ohno-Machado et al., 1998), ProForma (Sutton & Fox, 2003), as well as Decision Languages such as HL7 Gello and OMG DMN, and the HL7 Clinical Quality Language (CQL). These languages were not directly used, for various reasons including:
-
they do not support a clear distinction of ontic versus epistemic subject variables;
-
lack of an integrated plan / workflow formalism (all except DMN);
-
some are too procedural rather then declarative (Arden, GLIF);
-
current versions of some of these languages have been made specific to the HL7v3 RIM, a particular (and now obsolete) model of health information designed for message representation (GLIF 3.x, GELLO);
-
none of them support an integrated representation of the 'semantic stack' of process representation, decision logic, proxy data and data access;
-
the maintenance and support is unclear and/or limited.
Nevertheless, the design approach of Arden provided useful inspiration for the formalism described here, and indeed the Subject Proxy approach used in openEHR may be understood as a solution to the so-called 'curly braces problem' of Arden (Samwald, Fehre, de Bruin, & Adlassnig, 2012), i.e. the need to be able to bind rule logic to real-world variables. The ProForma language and underlying scientific analysis also has much to recommend it, and has been used as an important theoretical input to the specifications developed for openEHR. The HL7 CQL standard, although lacking a workflow language, provided useful insights including on temporal logic operators (originally described in Allen (1984)), data binding and general expression language design.
2.3. Relationship to Standards
The following table indicates the approximate correspondences between the openEHR process specifications and various published standards.
openEHR Formalism | HL7 standard | OMG standard | Other |
---|---|---|---|
CQL, FHIRpath? |
|||
CQL ELM |
DMN FEEL |
(numerous) |
|
(SDMN) |
The OMG group of standards are starting (as of 2020) to find use in the healthcare arena under the banner BPM+.
2.4. Theoretical References
Numerous publications have appeared over the last 30 years on process automation, decision support and related subjects, including within healthcare. A small number of seminal publications have been particularly valuable in the development of the openEHR Process-related specifications, including Rector Johnson Tu Wroe & Rogers (2001) and Hofstede van der Aalst Adams & Russell (2010).
The first of these presented an often-cited diagram capturing the existence and challenges of representation between the various elements needed in computable guideline technology, shown below.
The authors enumerated many challenges directly relevant to the specifications described here, including the following:
-
the need for a coherent, symbolic means of referring to real-world entities from guidelines to patient data sources (originally: Need for inferential abstractions; Ambiguity of operational meaning);
-
the need to abstract away differing concrete representations of subject data e.g. 'blood pressure' in back-end systems (originally: Differences in encapsulation and form of expression);
-
the need to be able to convert quantitative data values to ranges, e.g. blood pressure of 190/110 to 'elevated blood pressure';
-
computation of a subject value from underlying values via a formula, e.g. BMI from patient height and weight;
-
temporal predicates, e.g. 'rising blood pressure', 'being on steroids for 3 months' etc.
This paper presaged the notion of the 'vritual medical record', which itself may be seen as a precursor for the Subject Proxy concept used here.
3. Needs
This section discusses the requirements for a category of computational system that supports the execution of distributed, team-based, and long-running care processes by professional workers and subjects.
3.1. How Can IT Improve Clinical Care Process?
Naive approaches to the use of IT to support clinical care process generally assume that the benefits are obvious and automatic, and as a result, often fail to understand in what form computer support may be useful. An error common among IT professionals is to imagine that a clinical workflow system will itself perform the work currently performed by humans, i.e. to replace them. Even with the advent of AI-driven autonomous surgical robots and sophisticated decision support, this view is mistaken, since the process that executes within a workflow engine is not the same thing as the work undertaken by its various performers. Instead, an executable clinical process is better understood as a plan and related decision logic whose execution creates a sophisticated presencial helper within the cognitive space of each connected worker in the real world.
To understand the form a clinical planning system must take to be useful, we firstly need to understand how clinical work is performed in the real world.
The first aspect to consider is that a significant proportion of the activities of healthcare delivery are not clinical per se, but administrative and logistical, such as registering, booking, scheduling, transfers of care and so on. Nevertheless, the timing and conditions that govern appointments and transfers are unavoidably clinically driven, for example: the visits during routine ante-natal care; follow-up appointments post breast cancer treatment; determination of when to discharge a patient to long term nursing or home care. This tells us that there will be some representation of long-term planning in a clinical process support system, not just in administrative systems.
The second aspect to consider is that clinical professionals don’t need to be told how to do medicine. When the moment for performing clinical work arrives, with the exception of pure training situations, professionals already know what to do. What they may need is help with any of the following:
-
following care pathways and guidelines for complex conditions e.g. acute stroke, sepsis, complex childbirth; also guidelines that incorporate changing policy on the use of specific types of test whose costs and risks change over time (e.g. CT scan, MRI, certain drugs);
-
clinical check lists for multi-step procedures, including surgery, to prevent busy or tired personnel forgetting common steps;
-
reminders (that may be signed off) for critical actions in procedures not yet performed, particularly in team-oriented and busy environments (e.g. ED);
-
decision support to determine appropriate treatments, medications from among numerous and/or changing possibilities;
-
verification (signing off) of tasks performed for medico-legal reasons, process improvement, including process mining (see below).
In general, the above capabilities address three human fallibilities:
-
limited capacity to deal with complexity unaided, often denoted as the '7±2' problem, which refers to the limited number of variables that the majority of human beings can keep in mind at one time;
-
the inability to keep up with change (new medications, research etc); and
-
forgetting routine steps due to fatigue, rushing or an unexpected change of personnel in the middle of a procedure.
Automated support for the above would significantly aid the short-term, single clinician/patient situation, but much clinical care today is team-based, and also unfolds over a longer time than a single work shift or day. Therefore another important kind of help is needed:
-
coordination of:
-
team members across departments (via e.g. plan-generated notifications); e.g. acute stroke care;
-
workers undertaking care spanning shifts and personnel changes, e.g. multi-week chemotherapy administration;
-
longer term care, e.g. pregnancy, chronic disease monitoring.
-
A further clinical need which process automation can potentially address is reduction of documentation burden, which with the advent of EMR and other Health IT systems has become a major issue in recent years. In most healthcare delivery environments, clinical documentation is not synchronised with the process of the care delivery, but fitted in when possible, often after the fact. This creates a mental load on physicians, as well as sometimes forcing them to choose between more detailed documentation and spending time with the patient.
Process automation could also address the disconnect between work and documenting by supporting fine-grained, on-the-fly interaction with the EHR, such that as clinical work proceeds, documentation is also being done incrementally. This approach has the effect that all workers involved in the execution of a plan create documentation on the fly, rather than the senior clinician having to do all the documentation. When the work is done, the EHR is up-to-date, or very close to it, allowing all the involved clinicians to move very quickly to the next patient(s).
A further category of requirement for clinical process automation is training. In this case, the steps of a procedure may be second nature to an experienced clinician but for a trainee nurse or surgeon to whom much is new, a clinical guideline system would potentially represent even the most basic steps (e.g. cannulation, central line insertion, surgery preparation) to the trainee. Since being 'experienced' is not a binary state, but a continuum over one’s professional life, some level of training support is likely to be useful for any professional of any level of knowledge, e.g. to learn a newly developed surgical method, or revise a procedure not performed for some time. Training support therefore needs to be a flexible capability to be turned on or off at multiple levels of expertise, by any type of professional role, also based on work context.
A final and not immediately obvious benefit of process automation is the ability to record with reasonably good fidelity the steps and decisions taken by workers in the course of each procedure or episode of care. The resulting event logs provide a rich resource for process mining, a relatively recent discipline described in van der Aalst (2016), that uses the history of worker actions to discover how processes actually execute in the real world, with the aim of analysis and improvement of the original executable plan definitions.
The sections below elaborate on some of these topics.
3.2. Implementing Best Practices
Best practices in healthcare, especially for complex conditions and treatment pathways with co-morbidities, but also routine activities such as dialysis and angina diagnosis, are increasingly codified in the form of Care Pathways, Clinical Practice Guidelines (CPGs) and related artefacts published by professional colleges, universities, teaching hospitals, and national institutes. These artefacts are based on evidence-based and other kinds of research that have determined which specific approaches to care produce better and more repeatable outcomes for patients. They are usually published as PDF files or HTML websites containing a modicum of structural visualisation of decision pathways; they may contain hundreds of details to do with particular drugs, dosing, analyte levels, and investigational pathways.
One of the primary obstructions to the routine uptake of best practices publications is the inability to integrate them directly into the cognitive care environment of working clinicians. Their use relies on reading prior to care, and either memorisation or else use on a screen or in printed form at the point of care. Due to this basic difficulty, many guidelines are only used in a patchy way depending on individual clinician interest, spare time and psychological factors (Hoesing & International, 2016). Many of the major benefits are consequently not realised until they are incorporated into mainstream medical and nursing training, at which point they become the norm. The time lag for this to occur has been famously stated as being on average 17 years (Morris, Wooding, & Grant, 2011), and is thought to range from 10 to 25 years.
It has been recognised for some years that if best practice pathways and guidelines could be made computable in the form of plan and/or decision structures, they could be incorporated directly into clinical applications in use at point of care, greatly reducing the delay from research to use to potentially weeks or months rather than a decade or more via educational or manual dissemination routes.
3.3. Guideline and Pathway Authoring in Computable Form
If formalisation of natural language guidelines can succeed, a new possibility of major importance appears: the potential for the primary authoring of pathways and guidelines to be undertaken via tools that create formal expressions directly. This opens up further possibilities such as clinical guideline simulation, testing, and formal verification, which could revolutionise the both the production as well as the translation to point of care of best practices.
The ability to express best practice guidelines in a computable form thus lies at the heart of the requirements for automation of care process. The various kinds of artefacts are described in detail in Section 4.
3.4. Simulation and Training
One of the benefits that clinical process automation can bring is the ability to execute plans in a simulation mode. This can be done for a number of reasons:
-
during development of a guideline or pathway, as a means of 'debugging' it;
-
for training purposes for new personnel;
-
for training for experience personnel on rarely used or changed procedures;
-
to test alternative approaches to team structure, improve efficiency etc.
Computer-aided simulation of surgical procedures is not new (e.g. haptic feedback robotic systems with augmented / virtual reality visualisation are used to train surgeons in brain procedures), but is uncommon for longer running and team-based procedures e.g. complex childbirth, sepsis etc. However, medical simulation teaching environments do exist in which process simulation could be established, e.g. {https://www.ohsu.edu/simulation/about}[Oregon Health Sciences University (OHSU) simulation center^].
3.5. Long-running Processes
Orthogonal to the semantics of guidelines and pathways are the semantics of how automatable work plans relate to workers in the real world over time. A simple case is that when a plan is executed in an engine, worker(s) are attached by software applications or special devices, and detached at the completion or abandonment of the plan. This will work well enough for short running processes i.e. of minutes or some hours. Longer running processes are another question.
In general human workers are present for a shift or work day of a limited number of hours at a time, with a gap until the next appearance of the same worker. In healthcare, nursing and allied care professionals as well as house residents usually work on a shift basis, in which complete coverage of every 24 hour period is achieved over a series of shifts, while senior physicians and specialists are typically only present during 'normal working hours'. In the time domain of weeks and months, human workers go on holidays, leave job posts and clinics, and themselves die (being only human after all).
A similar kind of pattern, although usually with longer periods, applies to machines that function autonomously as workers (e.g. robotic surgery devices). This is because all machines need to be serviced and in the long term, obsoleted and replaced. Service patterns will be a combination of regular planned down-times and unplanned failures.
The general picture of worker availability within a facility is therefore one of repeating cycles of presence (shifts, work days, in-service periods) during normal at-work periods, punctuated by variable temporary absences for holidays, sickness, and downtime, as well as permanent absence. Worker availability for a given subject at a given moment is a subset of the overall availability within the facility, since any worker may be occupied with some subjects to the exclusion of others, including unplanned attendance (emergencies etc).
In contrast to this, the 'work to be done', whether a well-defined procedure (e.g. GP encounter, surgery) or open-ended care situation (diabetes, post-trauma therapy) will have its own natural temporal extension. This might fit inside a short period of a few minutes or a single shift or work day, i.e. a work session, during which the workers do not change. Anything longer will consist of a series of 'patches' in time during which the work of the plan is actively being performed - i.e. during encounters, therapy sessions, surgery, lab testing, image interpretation and so on.
A priori, healthcare systems, via the administrators, managers, and clinicians in each facility generally make concerted efforts to maintain continuity of care, e.g. by arranging of appointments to ensure that as far as possible, the patient sees the same care team members over time, and by personal efforts to ensure that each logical segment of care is completed in a coherent fashion (for example in antenatal care).
Nevertheless, a plan automation system cannot necessarily assume worker availability, or that it is guaranteed to cover the periods in time during which the patient needs attendance, although ICUs, surgical units etc would usually get close. An automatable plan representation will therefore need to explicitly incorporate the notion of allocation and de-allocation of workers to tasks (including in the middle of a task), as well as hand-overs between workers. This would imply for example, that a task within a plan cannot proceed until an appropriate worker had been allocated to it, which further implies that some basis for allocation may need to be specified. The YAWL language (Hofstede, van der Aalst, Adams, & Russell, 2010) for example supports various allocation strategies such as 'first available', 'most frequently used' and so on.
3.6. Cognitive Model
3.6.1. The Co-pilot Paradigm
Common to all of the categories of requirement described above is a general need that any planning / decision support system augment rather than replace the cognitive processing of workers, by providing judicious help when needed. In this view, the system acts like a co-pilot, and does not attempt to be the pilot. It may remind, notify, verify, answer questions and perform documentation, but always assumes that the clinical professionals are both the ultimate performers of the work as well as the ultimate deciders. The latter means that workers may at any time override system-proposed tasks or decisions. Similar to a car navigation system, a clinical co-pilot must absorb deviations from original plans and recompute the pathway at each new situation, as it occurs.
The co-pilot paradigm has direct consequences for formal representation of plans and decision-making, including:
-
the interaction between a worker and the guideline / decision support system might be very fine- or coarse-grained, i.e. the worker may ask the copilot for input frequently or infrequently;
-
a worker may treat computed inferences (i.e. rule results etc) as recommendations that may be overridden (usually with the ability to record justification); this implies a specific kind of interaction with a plan automation system unlike pure automatic computation (as would be used in an industrial process for example);
-
a worker may request the chain of logical justification of a particular rule result; this implies that rule execution must be done so that the execution trace is available for inspection.
3.6.2. Voice-based HCIs
One kind of technology that is becoming routine is voice-based human/computer interaction (HCI). Voice technology has become a useful convenience for using mobile phones while driving or interacting with home audio-visual systems, where it is replacing the remote control. It is likely to become the principle means of HCI in many clinical situations, since it achieves two things difficult to achieve by other means:
-
by replacing physical keyboard interaction with voice, it enables interaction with the system to occur in parallel, and therefore in real-time, with clinical work that typically already occupies the worker’s hands and eyes;
-
it largely removes the problem of maintaining the sterile field around a patient that would otherwise be jeapordised by multiple workers touching keyboards and touchscreens.
Voice control is also likely to be crucial to enabling a clinical process support system to operate as an intelligent co-pilot rather than an overbearing presence in the work environment, since it starts to emulate the normal conversational abilities of human workers, via which any principal worker may ask for help as needed, but also limit system intervention when it is not needed.
3.7. Activation of Plans, Guidelines and Decision Support
One of the basic challenges that emerges as soon as computable decision support, guidelines or planning are introduced to the workplace is how the appropriate artefacts from among possible candidates are activated. There are at least three ways this can happen:
-
via static linking of CPGs, plans etc to specific applications and forms, which are launched intentionally by the worker for each kind of work, e.g. specific type of patient visit;
-
via rules that execute when a particular application runs, to try to identify appropriate plans to use;
-
via a rule evaluator running in the background that executes on various events, e.g. data being committed to the EHR (e.g. test results), device data values, or simply on a timed basis.
The first of these is likely to be used with more comprehensive pathways and guidelines, such as ante-natal care, that have their a dedicated application or form within another application. The second approach normally limits guidelines activated to candidates matched to the type of patient or condition of the application in question, and might offer choices to the user. The third approach is normally used to run decision support guidelines designed to generate alerts for patients with specific risks, and might range from medication recommendations for patients showing evidence of hypertension to alerts for notifiable infections, such as methicillin-resistant staphylococcus aureus (MRSA) and Covid-19.
Mechanisms based on Event-Condition-Action (ECA) rules such as CDS-hooks are used to enable events in the clinical work environment to create requests to external CDS services and return recommendations. A well-known problem with injudicious launching of guidelines or rules is 'alert fatigue' due to numerous and/or incoherent alerts only weakly related to the patient. Uncontrolled alerting can adversely affect patient safety, since clinicians can easily miss the few important alerts that may occur.
Various requirements on computable representation of plans and guidelines follow from the above considerations:
-
care pathways, therapeutic guidelines and order sets need to include clinical indications, defined in terms of health conditions (e.g. having viral pneumonia), current medications or other evaluable criteria, which allow matching to subject state;
-
CDS (diagnostic) guidelines might include broad patient matching criteria (e.g. age, sex, being diabetic) rather than precise indications, and activation often relies only on the required input variables being available.
3.8. Integration with the Patient Health Record
General-purpose workflow formalisms and products do not generally assume the presence of a system whose purpose is to record information (e.g. observations, decisions, orders, actions) undertaken for the subject, beyond some direct record of the plan execution itself. However many tasks in healthcare plans involve the review and/or capture of complex data sets specific to the task at hand, which would naturally be recorded in the patient record. In order to make clinical plans efficient for their users, the formal representation of tasks needs to account for precise, unambiguous data sets and detailed action descriptions. For example a task whose short description is 'administer Cyclophosphamide, day 1' will have a detailed description as shown in the highlighted row of the following table:
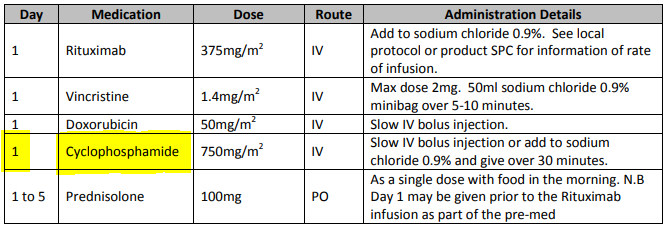
In an application, the dose will have been pre-computed based on patient body surface area. The administration description will usually be recorded in a structured way, e.g. {medication=cyclophosphamide; dose=1mg; route=IV; timing=30 mins; method=with 0.9% NaCl, …}
.
From a user perspective, if this information structure (in an appropriate unfilled template form) can be directly associated with the task within a plan in such a way as to enable easy filling in of the data and subsequent recording in the patient record, no further work is required to update the record at plan (or task) completion. Similar situations require display of specific data sets as part of performing a task. However, if patient record interactions cannot be tightly associated with tasks in a plan, plan automation may not significantly reduce clinician documentation burden, and may have limited value. Worse, if there is no ability to associate information retrieval and recording actions with their real world tasks, plan authors will be forced to create tasks within plans dedicated to these information system interactions. This will have the effect of greatly increasing the size of many plans while reducing their comprehensibility.
In an ideal realisation of healthcare process automation, the data sets would be standardised, and most likely part of the plan definition. However, for many practical reasons, data sets vary across environments, and a realistic approach to integrating data sets with plans needs to allow for both explicit declaration and anonymous referencing. The former may be used in environments that support detailed clinical data-set definitions (e.g. openEHR archetypes and templates, published HL7 FHIR profiles, Intermountain CEMs etc), whereas deployment in environments with mixed back-ends and legacy EMR systems will more likely require plan tasks to simply reference native EMR or other application UI forms.
3.9. Independence of Reusable Guidelines from Legacy HIS Environments
One of the hardest problems to solve historically with respect to computable guidelines and pathways has been how to author them so as to reference needed external data about the subject, but to do so independent of any particular back-end system environment. The general situation is that the data items, which we term subject variables, needed by a plan or guideline are populated from numerous kinds of back-end systems and products, including EMR systems, disease registers, departmental systems, research systems and increasingly, real-time devices. Each of these have their own data models, terminologies and access methods. Although there are standards for accessing such systems including standards from HL7 (HL7v2, CDA, FHIR), IHE (XDS), OMG, and IEEE these are themselves used in different forms and 'profiles', and are not used on all systems, particularly smaller research or practitioner-specific systems. Additionally, which data interoperability standards are in use in particular places changes over time.
In order to ensure computable plans and guidelines are independent of the heterogeneity of both back-end systems and ever-changing data standards, an approach is needed such that subject variables are declared symbolically within the computable representation, and are mapped to local system environments in a separate location, such as a dedicated service.
4. Care Process Artefacts and their Formalisation
Various kinds of potentially formalisable artefact commonly used in healthcare are implied by the needs described earlier, including the following:
-
care pathway: evidence-based plan of care for a complex condition;
-
clinical practice guideline (CPG): evidence-based protocol for a specific clinical task;
-
order set: 'template' set of combined orders for tests, medications etc relating to a condition;
-
care plan: descriptive plan for individual patient care, typically either oriented to in-patient care or post-discharge nursing care.
These artefacts do not constitute the totality of requirements of formal care process models, because they generally do not take into account the cognitive aspect of their use in care delivery by human professionals. The final inferences, decisions and actions of the latter will often use pathways and guidelines as a major input but only in the 'copilot' sense described above.
Nevertheless, the formalisation of the semantics of these artefacts is a major part of the overall semantics of the languages described here. Accordingly, what these are considered to consist of within the openEHR process architecture, and how they interrelate is described below.
4.1. Best Practice Artefacts
4.1.1. Clinical Practice Guidelines (CPGs)
Note
|
in openEHR specifications, the term guideline is used as a synonym for 'clinical practice guideline'. |
A commonly cited definition of a guideline is (emphasis added):
-
a systematically developed statement designed to assist health care professionals and patients make decisions about appropriate health care for specific clinical circumstances (Oxford Centre for Evidence-based Medicine).
A more recent version of this puts emphasis on the evidential basis:
-
Clinical guidelines are statements that include recommendations intended to optimize patient care that are informed by a systematic review of evidence and an assessment of the benefits and harms of alternative care options (Graham, Mancher, Wolman, Greenfield, & eds, 2011)
Guidelines are usually either:
-
therapeutic, i.e. oriented to describing a procedure e.g. intubation; medication dosing and administration; and management of a specific condition e.g. asthma, or;
-
diagnostic, such as for diagnosing various forms of angina.
Many diagnostic guidelines are relatively simple scores based on a number of patient variables, whose aim is to stratify risk into categories corresponding to type of intervention. Examples of guidelines include:
-
American College of Emergency Physicians (ACEP) Covid19 Severity Classification tool;
-
Australian Clinical Guidelines for the Diagnosis and Management of Atrial Fibrillation 2018 (Brieger, Amerena, Bajorek, & others, 2018).
4.1.1.1. Score-based Decision Support Guidelines
A very common kind of clinical guideline takes the form of a score, and consists of the following logical parts:
-
an input variable set;
-
one or more score functions of the input variables that generates a numeric value(s);
-
one or more stratification table(s) that convert score values to care classifications, e.g. 'refer to ICU' / 'monitor 24h' / 'send home'.
Although technically relatively simple, this kind of guideline can be very effective in changing clinical behaviour, primarily due to the fact that the score function and stratification table reflect the current evidence for the relevant condition. Such guidelines are usually executed in a single-shot fashion to generate recommendations for the clinician at the point of care. They are often visualised as a single form containing the input variables, the score and the resulting classification, with the input fields being populated from an EMR and/or manually fillable as required.
The openEHR GDL2 language is used to represent this kind of guideline, and implementations typically show interactive forms such as the following.
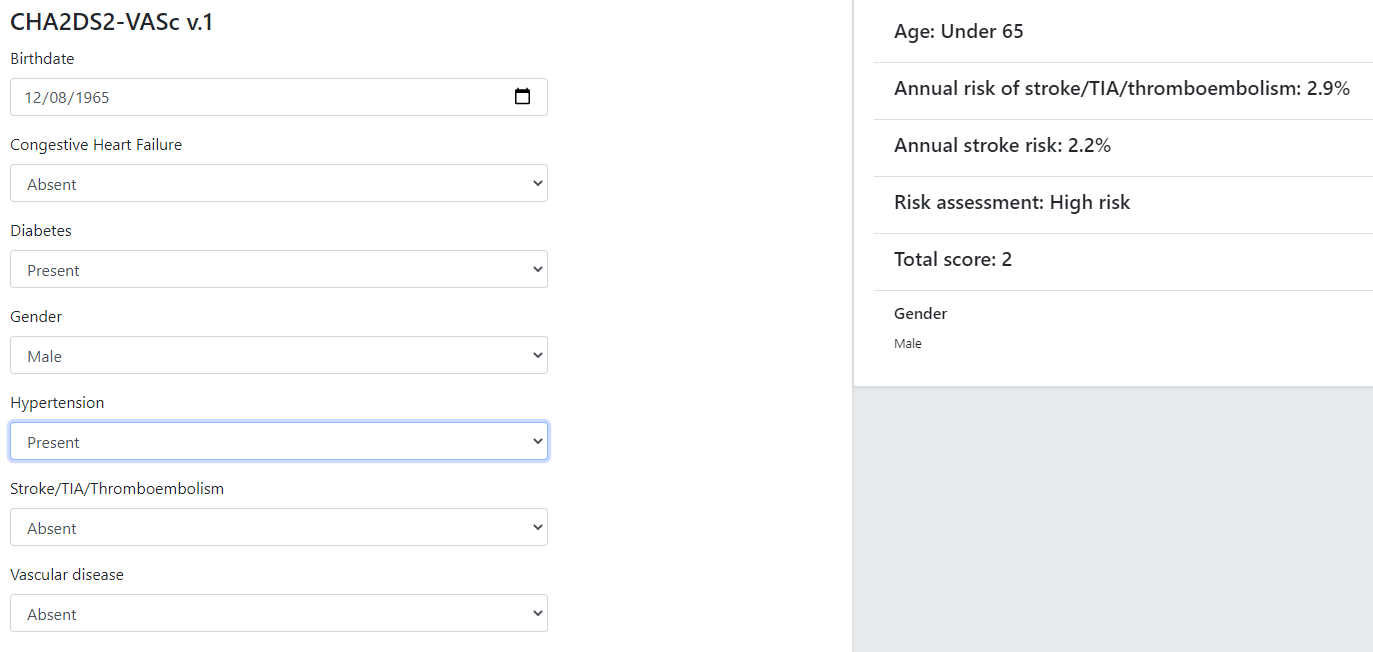
Some hundreds of similar guidelines are available in the openEHR GDL2 Clinical Models Repository.
4.1.2. Care Pathways
The notion of care pathway, also known by names such as 'integrated care pathway' (ICP) and 'critical care pathway', has various definitions in healthcare, such as summarised by the European Pathway Association (E-P-A) and many other authors and organisations. Based on these, we take a care pathway to be:
-
a structured description of complex care based on evidence, oriented to managing a particular primary condition;
-
patient-centric (rather than institution- or procedure-centric) and therefore multi-disciplinary and cross-enterprise.
A care pathway is thus a higher-level entity aimed at achieving a patient outcome, whereas a guideline is aimed at performing a more specific professional task. Consequently, care pathways typically refer to one or more guidelines that describe in detail how to deal with specific situations within the management of the condition (Schrijvers van Hoorn & Huiskes (2012), van Haecht de Witte & Sermeus (2007)). Care pathway examples include:
4.1.3. Order Sets
Within the above-described artefacts references to so-called order sets may exist. An order set is understood as:
-
a set of orders for diagnostic tests and/or medications and/or other therapies that are used together to achieve a particular clinical goal, e.g. the drugs for a particular chemotherapy regimen are often modelled as an order set;
-
potentially a detailed plan for administration of the items in the order set, which may be a fully planned out schedule of single administrations on particular days and times;
-
descriptive meta-data, including authors, history, evidence base, etc.
In most EHR/EMR sytems, the first item corresponds to a set of 'orders' or 'prescriptions', while the second is a candidate for representation as a formalised plan.
In the openEHR process architecture, an order set is considered to be an artefact of the same general form as a CPG, oriented to closely related orderable activities. It is therefore treated as a specific sub-category of plan artefacts, whose initial actions consist of a condition-specific set of orders with associated descriptive information. Administration actions may follow, within the same plan. Similarly to a care pathway or CPG, an order set may need to be modified for use with a real patient due to interactions or contra-indications, and any administration plan provided (perhaps as a template) may need to be copied and adapted for use in a larger patient-specific plan. Following this analysis, the question of formal representation of order sets is assumed to be solved via the same plan representation described for care pathways and CPGs.
4.1.4. Representation Challenges
Care pathways and guidelines are published based on studies performed on the available evidence base. This often occurs initially at a specific institution that is a recognised center of excellence for a condition (e.g. aortic dissection), or as the result of a wider research study focussed on a specific disease cohort (most rare diseases). Over time, successful guidelines and pathways find wider adoption and may migrate to a professional college or national institute for the long term. Regardless of the development history, there are a number of important issues that affect potential formalisation.
The first is the problem of partial coverage. There is no guarantee that any particular condition will have a published care pathway or guidelines for all of its subordinate activities. Coverage is likely to be partial, or sometimes completely absent. Consequently, the definition of a pathway for a particular patient (type) may have to be undertaken locally by institutions and/or simply achieved by 'old school medicine'. This implies that some automatable patient plans will be developed manually rather than from any existing pathway template.
The second issue is the problem of adaptation, which can be divided into two sub-problems. The first is that each pathway or guideline is designed to address one primary condition (sepsis, ARDS, angina etc) and will not generally be applicable unmodified to a real patient, due to patient specifics including co-morbidities, phenotypic specificities, current medications and patient needs and preferences. We might term this as a merge problem since it is essentially a question of arriving at a safe pathway for an actual patient that accounts for all of the patient’s current conditions (and therefore multiple applicable care pathways), medications and phenotypic specifics. Secondly, local practice factors such as formulary, local protocols, type of care setting (community clinic/hospital versus tertiary care centre/teaching hospital), availability and cost of imaging, drugs for rare conditions etc, will often constrain and/or modify any standard pathways or guidelines. We can understand this as a localisation problem.
A third issue is the practical challenge of there being multiple guidelines and pathways for the same purpose (i.e. diagnostic or therapeutic pathway), published by different institutions, including in different countries. Such guidelines may have been arrived at via different methods, evidence bases and may have variable coverage of the condition or procedure. They may also be expressed at differing levels of detail. We can think of this as a competition problem. Generally, the competition problem will be solved organisationally, e.g. by the institutional clinical quality board / group making choices, or even synthesising 'best of breed' versions from the existing candidates for a given use.
Assuming that the competition problem is solved within the local context, the merge problem will still usually occur with the consequence that more than one pathway may apply to a patient, e.g. one for chronic care and one for an acute complaint. There may be conflicts between the pathways - commonly in medications recommendations - but also between the pathways and the other patient specificities. Well-written pathways and CPGs usually include obvious contra-indications for medications (e.g. being on anti-rejection medications post transplant conflicts with some chemotherapy drug classes), common phenotypic features (e.g. being female, being allergic to taxanes), and patient history (e.g. being pregnant). There is no guarantee that any given pathway or guideline covers all possible conflicts, hence manual inspection, adjustment and customisation is almost always required. Localisation factors often means further modifications or constraints.
There currently appears to be little science on adaptation of guidelines, which means there is no obvious candidate method that might lead to automated or computer-aided adaptation. However communities such as the Guidelines International Network have working groups related to adaptation and localisation, which may lead to such methods in the future (Kredo et al., 2016).
For convenience, we term a care pathway- or guideline-like artefact for a specific patient, incorporating any necessary conflict resolution, merging and localisation, a patient plan.
With respect to the challenge of applying information technology to process-oriented care, key questions to do with published (natural language) pathways and guidelines are:
-
the extent to which they are formally representable, including contra-indications and conflicts;
-
how conflict, merge and localisation is solved to produce an automatable patient plan.
4.1.5. A Descriptive Model
We make a baseline assumption that guidelines and care pathways are essentially the same kind of entity in terms of structure, and are formalisable with the same model or language, with any differences (e.g. in goal or subject) handled by variable elements of the formalism. A survey of published pathways and guidelines shows that they consist of:
-
goal (pathway) or purpose (guideline);
-
indications, i.e. clinical pre-conditions for use;
-
plan: structured natural language statements describing a plan, i.e. a struconsisting of tasks (also known as activities), decisions (understood as a special kind of task), and wait conditions (enabling tasks to be situated in time or to be ready when certain events occur). Tasks and decisions may be sequential or concurrent, and which may relate to:
-
observations and assessments;
-
orders or order sets;
-
medication administration;
-
-
classification rules that convert a real-world value to a classification for the purpose of the guideline, e.g.:
-
SpO2 of 88% → 'critical' in a Covid19 assessment tool;
-
Systolic pressure > 160 mm[Hg] → 'high', in a hypertension guideline;
-
-
decision rules, in the form of logical statements, flowcharts, and tables, defining the primary logic of the artefact, e.g. a risk classification for a patient based on N subject variables.
Formalising such a structure primarily involves finding sufficiently powerful language(s) for the plan and logic (i.e. rules) parts. Following the general principle of separation of concerns, we assume that the languages for plan representation and rules definition are likely to be distinct, and indeed that plan artefacts and rules/decision modules are preferably separate and related by reference. This enables decision logic to be developed governed independently of particular plans, and prevents bad practices such as clinically significant rules being hidden inside plan definitions (typically on decision nodes).
Assuming this can be achieved, the second challenge then requires support within tools such that formal patient-level plans could be adapted in a fine-grained from existing pathways and CPGs and/or developed de novo when needed.
4.2. Care Plans
The care plan is a common artefact within clinical care, originating in nursing and relates to a specific patient rather than a condition. Definitions include a nursing-oriented definition from RN-central and one from the ISO Continuity of Care standard ('contsys'). From these we synthesise the following definition:
-
care plan - a dynamic, personalised plan, relating to one or more specified health issues, that describes patient objectives and goals, defining diagnoses and steps for resolution and monitoring.
Historically, a care plan has been a description of intended care that may be followed by relevant staff e.g. home-visit nurses. A patient may have more than one care plan, and the contents of a care plan may be informed by one or more care pathways and/or CPGs, or might be 'standard local practice'. A care plan may even be ad hoc in the case of a patient type with no well-described models of care available.
Within the openEHR process architecture, a care plan is considered a structured artefact whose contents are consumed by human actors, rather than being a directly automatable entity. It is assumed to include items such as:
-
identifier and purpose;
-
descriptive text;
-
potentially references to CPG(s) or care pathway(s) that apply, with any necessary modifications;
-
goals and targets;
-
relevant problems and diagnoses;
-
interventions: medication and other orders (and potentially order sets);
-
monitoring criteria / instructions.
A care plan may be formalised in the sense that the referenced CPG(s) and/or care pathway are formalised as a personalised patient plan (per above).
4.3. Formalisation
4.3.1. Conceptual Model of Guidelines
In the description of guidelines above, two of the key candidates for formal representation are plans and rules. Over some decades, the use of formal languages specifically designed for representing computable CPGs, often known as computer interpretable guidelines (CIGs), including Arden, Asbru, EON, PROforma and others (summarised in Sutton Taylor & Earle (2006)) has shown that plans and rules indeed emerge as the two main components, and each consists of certain conceptual elements. Not all of these languages agree in all details, nor support all concepts equally well (temporal operators for example), however the common set of general features can be used to inform a conceptual basis and nomenclature for the formal elements of CIGs, which we take to be as follows:
-
plans consisting of:
-
tasks, also known as activities;
-
decisions, understood as a special kind of task;
-
wait conditions, enabling tasks to be situated in time or to become ready when certain events occur;
-
-
logic modules containing:
-
conditions, i.e. simple Boolean-valued expressions referring to subject variables;
-
rules, i.e. more complex computations generating results of any type (quantity, coded term, etc) codifying the main decision criteria described in a guideline;
-
-
subject proxy, consisting of the set of subject variable declarations relevant to the rest of the logic.
An architecture based on these precepts would of course have to solve the question of referencing from plans to logic modules and from both of those to the subject proxy variables, as well as the semantics of run-time loading, execution and much else. The above just establishes the basic conceptual vocabulary for such developments. The following diagram illustrates these conceptual elements.
Some simple distinctions are thus made, for example that a decision is a kind of task (or activity) undertaken by a cognitive agent, whereas a rule is an algorithm for computing the values on which basis decision pathways are taken.
One of the important assumptions made here is that all conditions, rules and other decision computations (e.g. Bayesian logic, calls to AI services) are defined within logic modules, rather than being included within plans, as is the common practice for example in the use of most workflow formalisms (BPEL, BPMN, etc). This is to ensure all computable criteria, no matter how trivial-seeming (e.g. the expression systolic_blood_pressure >= 160
) are represented and documented in one place, and in a form that may be understood and maintained by the domain experts and guideline authors.
4.3.2. General Vision
The clinical artefacts described above may be classified as follows, for the purposes of potential computable representation:
-
automatable artefacts: care pathways, guidelines, order set administration plans;
-
structured artefacts: care plans.
Following the preceding section, automatable artefacts are assumed to consist of at least three kinds of element:
-
descriptive: structured description, identification etc;
-
plan: a representation of tasks, events and decision points (also known as workflow);
-
decision logic: subject variable declarations and rules.
None of the above artefacts acts directly as an executable plan for a specific subject (i.e. patient). Care pathways and guidelines each relate to a single isolated condition or procedure, whereas the general situation for a real patient is multiple conditions plus phenotypic specificities (e.g. allergies) plus current situation (e.g. being pregnant) plus non-clinical elements (e.g. patient preferences, type of health plan cover etc). Adaptation and merging is in general unavoidable.
Although there is no commonly recognised term for an patient-specific CIG, we assume its existence and term such an artefact a patient plan for convenience, and make the assumption that for the purposes of formal representation it is a combination of:
-
a care plan that describes the intended care approach (may be minimal in some circumstances, e.g. emergency);
-
a potentially executable pathway of the same formal representation as a care pathway or guideline, but whose content is adapted from relevant automatable CPGs and/or care pathways, where available.
Since a computable patient plan may originate from a full care pathway, such as for complex pregnancy, or a simple guideline, such as CHA2DS2–VASc, it may express any level of clinical detail.
The various clinical artefacts described above and related computational entities, along with their relationships, can be visualised as follows.
In the diagram, the term computer-interpretable guideline (CIG) is used to denote any formal representation of plan and related decision logic that could be executed by an appropriate engine. A CIG can thus be used to represent both condition-specific guidelines, care pathways as well as a patient plan. For the latter, it is assumed that the executable representation of a care plan may be included, where one exists.
Entities shown with dotted lines are not assumed to exist in all real world clinical situations. That is, care may be being provided for a patient for which no published care pathway is available, and only limited published guidelines. This would imply no or limited availability of condition-specific executable plans for use in constructing an executable patient plan. Nevertheless, the latter could be constructed de novo, rather than by adaptation of library pathways or guidelines.
4.3.3. Separation of Concerns
As implied by the analysis of Rector Johnson Tu Wroe & Rogers (2001), the formally represented pathways and guidelines shown on the right hand side of Figure 5 have important relationships with both terminologies and data sources such as the patient record. Some of the requirements described above - independence of guidelines from legacy HIS back-ends, and also the need for a high-level language of guideline authoring that enables 'smart' subject variables such as 'highest systolic pressure over previous 2 weeks' and 'oxygen saturation, no older than 1 hour', as well as functions of base variables, such as Body Mass Index (BMI, a function of height and weight), lead naturally to a greater separation of guidelines from information models of the back-end systems.
The general approach taken by the openEHR specifications is to treat smart subject variables as a first order concern termed here data enrichment. We also recognise a more contemporary view of patient record systems, devices and intermediate interoperability standards designed to retrieve data from such systems in a more homogenous way than in the original version of the Rector paper (from 2001). The conceptual view that results is shown below in a modified version of the original figure.
In the above, a subject proxy model is introduced that represents one or more subject variables, including basic measurable values (e.g. heart rate), functions of other values (e.g. BMI), and computed ranges (e.g. conversion of systolic pressure = 165 to 'very high' range), interval averages and so on. It is assumed that each guideline or pathway (or related collections) have their own specific set of subject variables. A subject proxy is therefore not a fixed global model like the older 'virtual medical record' notion, but instead a limited virtual view of the subject relevant to some guideline.
5. Deployment Paradigm
5.1. Separation of Worlds
As soon as the notion of planning is assumed, we enter the workflow space, and it becomes essential to describe the intended relationship of humans and machines in the work environment. This is due to the fact that any description of planned work acts as a set of instructions to actors intended to perform the tasks. Since the instructions (task plans) will be represented in the IT layer and the performing actors (generally human, although they may also be autonomous devices or software applications) exist in the real world, an account of the interaction between the computing environment and the real world is required.
Firstly, we distinguish the following entities in the work environment:
-
computing environment:
-
executable plan definition: a reusable definition of work to be done, consisting of tasks, potentially standardised according to a guideline or protocol;
-
executable plan instance: a run-time instance of a task plan, potentially with local variations, created for execution by an actor or actors;
-
-
real world:
-
performing actor: an autonomous human, machine or software application that performs tasks in the real world as part of a procedure designed to achieve a goal;
-
In real-world environments, the actors are not passive recipients of commands from a computer application implementing a plan, but are instead active agents who normally work together to perform a job. Working together involves peer-to-peer communication, coherent sequencing of tasks and so on. A workflow application provides help by maintaining a representation of the plan, and a representation of its progress in execution. It is immediately apparent that the application’s idea of a given plan execution and the real world state of the same work are not identical, and in fact may be only approximately related. For example, the computable form of the plan might only include some of the tasks and actors at work in the real world. There are in fact two workflows executing: a virtual workflow and the real world one, and there is accordingly a challenge of synchronisation of the two.
There is also a question of the nature of the communication between the workflow application and the real world actors, which we can think of as consisting of:
-
commands: signals from the plan system to a real world actor to do something;
-
notifications: signals to and from the plan system and the real world actors on the status of work, e.g. 'new work item', 'item completed' etc;
-
data: data collection from actors and presentation to actors from the system.
This view of the environment can be illustrated as follows.
5.2. Distributed Plans
There is a potentially complicated relationship between IT environments in which computable plans execute and the work-places where the human actors are found. This is because 'systems' in a concrete sense are part of IT installations owned by organisations that may or may not be the employers of the workers, while plans may logically span more than one distinct work-place. For example a clinical plan for diagnosing and resolving angina may contain steps that are performed by:
-
an emergency department (part of a hospital);
-
a general practitioner (in a separate health clinic);
-
a cardiologist (within a hospital, possibly a different one to the original ED attendance);
-
a radiology department (usually within a hospital, possibly also different, for reasons of availability, machine type etc).
These various healthcare facilities almost certainly have their own IT systems within a managed environment and security boundary, with some possible sharing of systems among some facilities. Consequently, where the notional clinical plan executes in the real world doesn’t usually cleanly correspond to one IT system in which a plan engine can execute it.
On the other hand, in an ideal environment with regional patient-centric hosted services (shared EHR etc), accessible by all healthcare facilities the patient visits, the logical locus of a plan’s activities - the various HCFs taken together - correspond 1:1 with a location where the plan can be executed by an engine, i.e. the regional system.
The following figure illustrates different possible relationships between a logical plan definition whose work is performed by actors in different enterprises, and the location of plan engines where such a plan may logically be executed. The particular arrangement shown has one enterprise (on the left) with its own separate IT (typical for most hospitals today) and three other enterprises that share regional health IT services relevant to the patient record and plan execution (they probably have their own private IT for more mundane purposes as well of course). The plan is shown as having tasks that are to be performed at all four of the enterprises.
The diagram implies a scheme in which the same plan might be executed in multiple places, presumably with synchronisation, however this is not posited as a requirement. The only hard requirement is that there is some means of enabling the various parts of a plan to execute in the various work-places required.
One of the challenges in such distributed work environments, which are the norm in healthcare, is the ownership, creation, maintenance and sharing of plan definitions that implicate workers across enterprises.
6. Conceptual Architecture
6.1. Overview
Within Figure 5, CIGs (computer-interpretable guidelines) are shown as the principal computable artefact of interest for the development of automatable clinical plans. This section describes the conceptual architecture of this artefact in some detail.
The primary formal elements of (CIGs) are the plan, i.e. a structured representation of tasks to be performed including decision points, and the decision logic, whose rules provide values for decisions, as well as declarations of subject variables. These are respectively denoted Plan Definition (or just 'plan') and Decision Logic Module (DLM).
Plans are formally defined by the openEHR Task Planning model, and visualised (including for authoring purposes) in Task Plan Visual Modelling Language (TP-VML). DLMs are written in the openEHR Decision Language (DL), which is a high-level syntax.
Both of these artefacts contain references to subject variables, i.e. ontic (real-world) subject state, such as date_of_birth, weight, systolic_BP and so on. Some of these variables are unchanging in time (e.g. date of birth; sex) or nearly so (height, in adults; type 1 diabetic status), while others are time-varying and may need to be tracked over time. Tracking subject variables over time might entail near real-time (i.e. seconds, minutes) update from measuring devices or simply repeated querying for routine measurements over time from the patient record. A collection of subject variables relevant to plans and DLMs is termed a Subject Proxy, i.e. a proxy representation of a real world subject (such as a patient) for use by plans, DLMs and other applications.
In order to execute a plan and its related logic, a means of populating a subject proxy is therefore required. This is termed a Subject Proxy Service. Within such a service, bindings to particular source sytems (queries, API calls etc) are installed, which are denoted here Data Access Bindings (DABs). The following diagram illustrates these conceptual artefacts.
6.2. Plans
In the above, the top box (pink) illustrates a plan definition, used to express a structured set of tasks. In openEHR these are expressed as Work Plans, defined by the openEHR Task Planning specification. Work plans are used for various scenarios, including:
-
long term management plans unfolding over a time period in which personnel constantly change, and the plan is the main record of work done / to do;
-
highly detailed actions defined by clinical pathways for complex conditions such as sepsis, where the complexity level is beyond unaided cognitive capacity;
-
reminders and checklist items for basic actions that are sometimes missed or forgotten due to busy workplace and fatigue;
-
actions requiring sign-off;
-
coordination of workers in a distributed team;
-
actions that result in recording something in the EHR;
-
actions of varying levels of granularity that are needed in a training mode.
A Work Plan is a complete plan with an associated goal and indications (i.e. clinical pre-conditions), and consists of one or more Task Plans, each being for one performer. The inner structure of a Task Plan consists of Task Groups, various kinds of Tasks (dispatchable and performable) and event wait states. The following illustrates a simplified Work Plan for acute stroke management.
Using this model, plans for any of the above situations can be defined by domain experts using dedicated graphical tools. Work Plans are executed by a plan engine, which connects with workers (generally human, although software agents and autonomous devices may also perform tasks) and acts as a co-pilot for each one, reminding them of tasks and relevant details according to the worker’s process. It also manages coordinating notifications and commits and retrieval to and from the EHR. In this way, it can convert disparate workers with weak communication into an integrated, coordinated team.
The Task Planning model supports various fine-grained mechanisms common in workflow languages, including: five types of task; a task life-cycle that supports completion, cancellation, and abandonment; repeat blocks; parallel and sequential task execution; AND, XOR and various kinds of OR join; wait states triggered by external, calendar-based and internal events; blocking and non-blocking execution threads; condition-gated exception task plans and ad hoc task insertion. Some of these features are visible in the following plan, which is for chemotherapy administration (full version).
One of the key formal elements of a Work Plan is decision points (green nodes in the plan diagrams above), where plan branching occurs, based on conditions and rules that ultimately depend on subject variables. In the openEHR architecture, all such decisional logic is expressed in dedicated Decision Logic Modules (DLMs), rather than within the plan. This ensures all decision conditions are treated as first order knowledge artefacts, rather than being hidden as (possibly duplicated) ad hoc expressions within the plan, where they are difficult to find and maintain.
6.3. Decision Logic Modules (DLMs)
In the conceptual model, the box on the lower right (green) represents decision logic modules (DLMs), which express the rules, decision tables etc that encode the decision logic of plans and guidelines. DLMs are used to represent the simplest Boolean conditions such as systolic_blood_pressure >= 140 mm[Hg]
used in plans, as well as complex chained logic representing stand-alone guidelines, scores and other rule-based clinical entities. To do this, they must declare subject variables needed for computing the rules and/or needed by client Work Plans or other applications.
The openEHR decision logic language may be characterised as a function-oriented logic that typically represents deductive inferences, such as clinical classification of patients (including diagnosis), based on input variables representing known facts about the subject (i.e. patient). These include results obtained from real world observation, measurement, imaging etc, as well as previous confirmed diagnoses and records of previous procedures. Other kinds of reasoning may be used as well, including Bayesian and other statistical and Artificial Intelligence (AI). In the latter case, DLM functions make calls to appropriate specialised services.
DLMs are written in the openEHR Decision Language (DL) consists of various formal elements, including:
-
input variables: declarations of subject-related variables that are referenced within the conditions and rules;
-
conditions: Boolean-valued simple rules based directly on subject variables;
-
rules: complex rules generating non-Boolean values;
-
rule-sets: collections of rules that operate on a common set of input variables to generate a common set of output values; may be represented as 2-dimensional decision tables;
-
output variables: intermediate rule results that may be inspected by calling components.
Conditions, rules, rule-sets and other inference-generating structures that may include them constitute fragments of knowledge that need to be able to be authored and change-managed independently from contexts that use them, rather than being directly written into (say) if/then/else logic chains as a programmer would typically do. This specification accordingly provides a representational form for such logic, along with mechanisms that connect them to data access services (e.g. EHR) and also enable them to be invoked by user contexts (e.g. workflow engines).
An extract from the DLM corresponding for the RCHOPS chemotherapy plan shown above is as follows (full version)
dlm RCHOPS21 language original_language = <[ISO_639-1::en]> description original_author = < ["name"] = <"Dr Spock"> ... > details = < ["en"] = < purpose = <"NHS CHOPS-21 chemotherapy guideline ...."> ... > > use BSA: Body_surface_area preconditions has_lymphoma_diagnosis reference rituximab_dose_per_m2: Quantity = 375mg ... cycle_period: Duration = 3w ... input -- State has_lymphoma_diagnosis: Boolean input -- Tracked state staging: Terminology_term «ann_arbor_staging» currency = 30 days time_window = tw_current_episode neutrophils: Quantity currency = 3d ranges = ---------------------------------- [normal]: |>1 x 10^9/L|, [low]: |0.5 - 1 x 10^9/L|, [very_low]: |<0.5 x 10^9/L| ---------------------------------- ; ... rules -- Conditions high_ipi: Result := ipi_risk ∈ {[ipi_high_risk], [ipi_intermediate_high_risk]} rules -- Main | | patient fit to undertake regime | patient_fit: Result := not (platelets.in_range ([very_low]) or neutrophils.in_range ([very_low])) doxorubicin_dose: Quantity Result := doxorubicin_dose_per_m2 * BSA.bsa_m2 * case bilirubin.range in =================== [high]: 0.5, [very_high]: 0.25, [crit_high]: 0.0 =================== ; ... | | International Prognostic Index | ref: https:|en.wikipedia.org/wiki/International_Prognostic_Index | ipi_raw_score: Integer Result.add ( --------------------------------------------- age > 60 ? 1 : 0, staging ∈ {[stage_III], [stage_IV]} ? 1 : 0, ldh.in_range ([normal]) ? 1 : 0, ecog > 1 ? 1 : 0, extranodal_sites > 1 ? 1 : 0 --------------------------------------------- ) ipi_risk: Terminology_code Result := case ipi_raw_score in ======================================= |0..1| : [ipi_low_risk], |2| : [ipi_intermediate_low_risk], |3| : [ipi_intermediate_high_risk], |4..5| : [ipi_high_risk]; ======================================= ; terminology term_definitions = < ["en"] = < ["paracetamol_dose"] = < text = <"paracetamol dose"> description = <"paracetamol base dose level per sq. m of BSA"> > ["chlorphenamine_dose"] = < text = <"chlorphenamine dose"> description = <"chlorphenamine base dose level per sq. m of BSA"> > ... > >
6.4. Subject Proxy
Plans and decision logic necessarily require a way of defining and expressing their input variables. This is not just a question of creating typed variables, but of their semantics. The 'variables' used in plan tasks (e.g. for display) and DLM rules represent an ontic view of the subject, that is, as close as possible to a true description of its state in reality. For example, a rule for inferring atrial fibrillation and other forms of arrhythmia may refer to the input variables heart_rate
and heart_rhythm
. The meaning of these variables is that they represent the real heart rate and rhythm of the patient, rather than being just any heart rate, e.g. from a measurement 3 years ago recorded within a particular EMR system. Similarly, a variable is_type1_diabetic
represents a proposition about the patient in reality.
To make decision logic comprehensible to (and therefor authorable by) domain experts, subject variable names need to be close to the language of the domain, for example is_type1_diabetic
and has_family_history_of_breast_cancer
are things a clinical professional directly understands. Semantically, they tend to be highly precoordinated forms of more technical representations, e.g. problem_list.contains (type = 73211009|diabetes mellitus|, status=confirmed)
which should of course be hidden in implementations.
Conceptually, the collection of subject variables of interest to a plan or DLM is a subject proxy, i.e. a (generally partial) proxy view of a subject in reality, such as a real patient. Accordingly, in Figure 9, two subject proxies are shown, attached respectively to the plan definition and the DLM, i.e. in the application execution context. These proxies maintain copies of variables needed by the executing plan and its logic modules. The proxies are connected to a Subject Proxy Service, which extracts data from back-end systems and other sources, and updates the proxies over time.
6.5. Subject Proxy Service
Extraction of subject state from its sources is managed by a Subject Proxy service. Data Access Bindings (DAB) are required within the service to extract data from specific data sources and repositories (i.e. via specific queries, APIs etc), such as patient health records, lab systems and monitoring devices. Where data is not available from these sources, users may be requested to provide it.
The Subject Proxy Service performs a number of jobs, which taken together, have the effect of 'lifting' data from the typically complex IT environment, and converting it to a clean representation of specific subject attributes relevant to specific applications, including CIGs and Decision Support. These jobs are described below.
6.5.1. Semantic Reframing: from the General and Epistemic to the Ontic and Use-specific
The relationship between guidelines and data exhibits a number of semantic characteristics that lead to the concept of the Subject Proxy as an independent interfacing service.
In order to define a care pathway or guideline (possibly adapted into a patient-specific care plan), various subject subject variables and events are needed. Since guidelines are specific to purpose, the number of variables is typically low, and for many simpler guidelines, as few as three or four. Many guidelines need access to common variables such as 'sex', 'age', basic clinical classifiers such as 'is diabetic', 'is pregnant' and then a relatively small number of condition-specific variables representing patient state (e.g. 'neutrophils', 'ldl') and specific diagnoses (e.g. 'eclampsia', 'gestational hypertension'). A guideline of medium complexity, such as for RCHOPS (non-Hodgkins lymphoma) chemotherapy needs around 20 variables, and a complex guideline such as for sepsis might need 50 - 100.
These small numbers are in contrast to the total number of distinct types of data point that will be routinely recorded for an average subject over long periods and relating to all conditions, which is in the O(1k) range, or the number of such data points recorded for a population, e.g. all inpatients + outpatients of a large hospital, which is O(10k). The latter corresponds to the variety of data that a general EMR product would need to cope with. The 'data sets' for specific guidelines are thus small and well-defined in comparison to the data generally captured within a patient record over time, and thus candidates for encapsulation.
Data set size is not the only distinguishing characteristic of a computable guideline. Where variables such as 'systolic blood pressure', 'is diabetic' and so on are mentioned in guidelines, they are intended to refer to the real patient state or history, i.e. they are references to values representing ontic entities, independent of how they might be obtained or stored. This is in contrast with the view of data where it is captured in health records or documents, which is an epistemic one, i.e. the result of a knowledge capture activity. Consequently, a query into a departmental hospital system asking if patient 150009 is diabetic, indicates that the patient is diabetic in the case of a positive answer, but otherwise probably doesn’t indicate anything, since the full list of patient 150009’s problems is often not found in departmental systems.
A query into any particular epistemic resource, i.e. a particular database, health record system or document only indicates what is known about the subject by that system. A true picture of the patient state can be approximated by access to all available data stores (e.g. hospital and GP EMR systems), assuming some are of reasonable quality, and is further improved by access to real-time device data (e.g. monitors connected to the patient while in hospital, but also at home). The best approximation of the ontic situation of the patient will be from the sum of all such sources plus 'carers in the room' who can report events as they unfold (patient going into cardiac arrest), and the patient herself, who is sometimes the only reliable origin of certain facts.
This epistemic coverage problem indicates a need which may be addressed with the Subject Proxy, which is to act as a data 'concentrator', obtaining relevant data from all epistemic sources including live actors to obtain a usable approximation of true patient state. This is a practical thing to do at the guideline / plan level by virtue of the small sizes of the variable sets. The data concentrator function is described in more detail below.
Comprehensive coverage of all possible sources is not the only problem to solve in order to define variables for use in guidelines and plans. In formal terms, symbolic references appearing at different levels in the environment have different semantics. Within the EHR system S1 for example, a generic API call has_diagnosis(pat_id, x)
has the meaning: 'indicates whether patient P is known to have diagnosis x, according to S1'. However, within a guideline related to pregnancy, a variable is_diabetic
defined in a Subject Proxy is more convenient, and is intended to represent the true diabetic state (or not) of the patient. The Subject Proxy Service thus not only has the effect of data concentration in order to extract a true ontic picture of the subject, but it reifies technical data access calls into ontic variables, specific to the guideline. In some cases, such variables might have pre-coordinated names such as previous_history_of_eclampsia
, combining a temporal region with a substantive state.
6.5.2. Manually Reported and Missing Data
A Subject Proxy acts as a data concentrator, providing a single interface to all available sources of information about the subject. In a typical in-patient or live-encounter (e.g. GP visit) situation, these include:
-
the EMR system providing the institutional patient record;
-
any shared (e.g. regional or national) EHR system providing e.g. summary and/or emergency data;
-
devices attached to the patient, e.g. vital signs, pulse oximeter etc.
In many cases, a variable required by an application, e.g. sufficiently recent patient weight, is not available from the EMR/EHR or from any other source. This is a common problem in all decision support environments, and the usual solution is that an application window is displayed to ask the clinician for the data directly. This may be entered (e.g. after weighing the patient or asking the patient for his last weight), saved into the EMR, and the original request retried. Traditionally, this data request 'loop' has been engineered into either the main EMR application or into the decision support component. It is however a general problem and can be conveniently solved in a generic way using the Subject Proxy.
Further, there are some subject state variables and particularly events that are only available 'live' from clinicians working with the patient, e.g. state of consciousness, occurrence of a post-heart surgery heart attack (requiring emergency cardiac shock and/or re-sternotomy), haemorrhage during childbirth etc. Such events can only be realistically asserted 'in the room' by a clinician, potentially via a voice interface.
Consequently, we can say that the following constitute two more routine data sources for a Subject Proxy:
-
just-in-time UI capture of missing data;
-
manually-reported events 'in the room'.
The effect of data concentration in the Subject Proxy is that the plan, decision support, and all other applications can rely on a single location to obtain patient state and events, even where the relevant underlying data are not (yet) available in source systems. Additionally, 'live' data obtained by such methods may be written to the relevant EMR and/or EHR by the Subject Proxy, removing the problem of other applications having to make ad hoc writes, following ad hoc data capture.
6.5.3. Type Conversion
A natural consequence of obtaining data from multiple sources is that the data will be instances of different concrete models (e.g. HL7 messages, documents and FHIR resources; openEHR query results; proprietary EMR data etc). It is also the case that the requesting plan-based and decision-support applications can work effectively with a relatively stripped down system of data types and limited structures. The latter is due to the fact that although data tend to be captured in larger structures such as full blood panels, full vital signs data sets and so on, guidelines and plans tend to require only specific lab analytes (e.g. troponin for investigating possible heart attack) and vital signs, e.g. systolic blood pressure (no need for diastolic pressure, patient position or other details).
The consequence of this is that the type system required at the Subject Proxy level may be significantly simplified compared to the type systems and structures in which data are originally captured. The use of subject proxy variables as the interface for decision support and plan applications to back-end systems greatly simplifies the artifacts needed in the latter components.
6.5.4. The Temporal Dimension: Currency
Another common problem traditionally handled by individual applications, including decision-support, is the currency of data, i.e. its 'recency'. Some variables such as body height are sufficiently current even when measured years earlier, while others such as oxygen saturation and heart rate need to be less than say 15 minutes old to be useful. To obtain valid values, applications often implement a scheme based on polling, automated server-side 'push' query execution, publish-subscribe or other mechanisms to obtain current data. None of this funcionality can really be avoided, but the Subject Proxy provides a single place to locate it, such that client applications simply access the SPO variables they need, and the SPO takes care of the update problem.
7. Architectural Use-cases
The formal artefacts described above may be deployed in different ways to achieve various goals.
7.1. Plan-oriented Systems
Many applications are designed around plans as the organising principle, such as complex medication administration (e.g. multiple cycles of multi-drug chemotherapy), team-based acute stroke response, ante-natal care and chronic condition management. In such cases, the system or application architecture will typically resemble the following.
7.2. Guidelines and Decision Support
Another major category of clinical process IT is guideline-based decision support. In this case, DLMs are used to express potentially complex guidelines to evaluate e.g. risk of acute events (heart attack, stroke etc). They are usually evaluated in an event-based manner, being triggered by e.g. commits to the EHR, or by a family practitioner opening the patient record for a particular patient.
References
Allen, J. (1984). Towards a general theory of actions and time. Artificial Intelligence, 23(2), 123–154.
Brieger, D., Amerena, J., Bajorek, B., & others. (2018). NHFA CSANZ Atrial Fibrillation Guideline Working Group: Australian Clinical Guidelines for the Diagnosis and Management of Atrial Fibrillation. Heart, Lung and Circulation, 27(10), 1209–1266. Retrieved from https://www.heartlungcirc.org/article/S1443-9506(18)31778-5/pdf
Graham, R., Mancher, M., Wolman, D. M., Greenfield, S., & (eds), E. S. (2011). Clinical Practice Guidelines We Can Trust (Institute of Medicine). Washington, DC: The National Academies Press.
Hoesing, H., & International, J. C. (2016). Clinical Practice Guidelines: Closing the Gap Between Theory and Practice. Retrieved from https://www.elsevier.com/__data/assets/pdf_file/0007/190177/JCI-Whitepaper_cpgs-closing-the-gap.pdf
Hofstede, A. H. M. T., Aalst, W. M. P. van der, Adams, M., & Russell, N. (2010). Modern Business Process Automation: YAWL and its Support Environment. Springer-Verlag.
Kredo, T., Bernhardsson, S., Machingaidze, S., Young, T., Louw, Q., Ochodo, E., & Grimmer, K. (2016). Guide to clinical practice guidelines: the current state of play. International Journal for Quality in Health Care, 28(1), 122–128. Retrieved from https://doi.org/10.1093/intqhc/mzv115
Morris, Z. S., Wooding, S., & Grant, J. (2011). The answer is 17 years, what is the question: understanding time lags in translational research. Journal of the Royal Society of Medicine, 104(12), 510–520. Retrieved from https://doi.org/10.1258/jrsm.2011.110180
Ohno-Machado, L., Gennari, J. H., Murphy, S. N., Jain, N. L., Tu, S. W., Oliver, D. E., Pattison-Gordon, E., et al. (1998). The GuideLine Interchange Format - A Model for Representing Guidelines. J Am Med Inform Assoc, 357–372.
Rector, A., Johnson, P., Tu, S., Wroe, C., & Rogers, J. (2001). Interface of Inference Models with Concept and Medical Record Models (pp. 314–323). Retrieved from https://www.researchgate.net/publication/221450288_Interface_of_Inference_Models_with_Concept_and_Medical_Record_Models
Samwald, M., Fehre, K., Bruin, J. de, & Adlassnig, K.-P. (2012). The Arden Syntax standard for clinical decision support: Experiences and directions. Journal of Biomedical Informatics, 45, 711–718. Retrieved from https://www.sciencedirect.com/science/article/pii/S1532046412000226
Schrijvers, G., Hoorn, A. van, & Huiskes, N. (2012). The care pathway: concepts and theories: an introduction. In International journal of integrated care.
Sutton, D. R., & Fox, J. (2003). The syntax and semantics of the PROforma guideline modeling language. J Am Med Inform Assoc., 10(5), 433–443. Retrieved from https://www.ncbi.nlm.nih.gov/pmc/articles/PMC212780/
Sutton, D. R., Taylor, P., & Earle, K. (2006). Evaluation of PROforma as a language for implementing medical guidelines in a practical context. BMC Medical Informatics and Decision Making, 6. Retrieved from https://bmcmedinformdecismak.biomedcentral.com/articles/10.1186/1472-6947-6-20